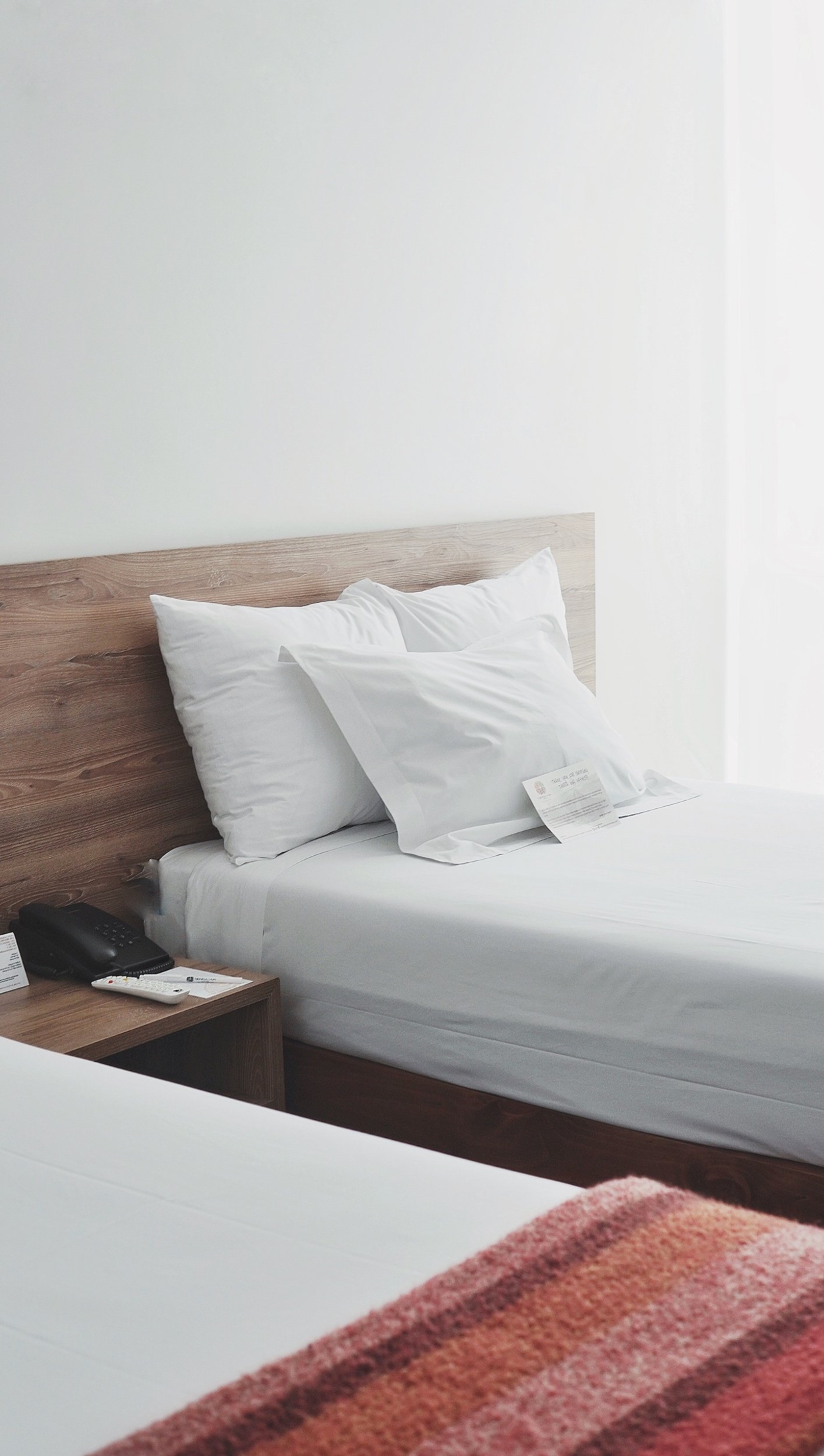
Search Results Page Hotel Recommendations:
utilizing AI to improve recommendation systems and alleviate decision fatigue in users.
Reimagined with ML & AI
UI Design
6 weeks
@ tiket.com
ℹ️ Context
tiket.com is one of the largest and most comprehensive online travel companies in Indonesia providing flight, train, accommodation booking, event ticketing, car rental, and other travel essentials
This redesign was done as a capstone project completed over the course of 10 weeks by a group of three interns. We were assigned to come up with an explorative solution to the main question:
How can the mobile interface be improved to maximize the capabilities of the already existing Machine Learning / Artificial Intelligence systems at tiket.com?
In providing a solution to this, we aim to in turn (1) improve user experience and (2) retain user engagement.
💡Background
1. Understanding Machine Learning (ML) / Artificial Intelligence (AI), and its capabilities in the OTA industry.
After doing our desk research, we discovered that a majority of online travel agencies utilize AI for a better user experience, particularly for curating recommendations for users.
Users are constantly looking for personalized content. (Bozhuk et al., 2020)
(This research studied the current conditions of the tourism industry from consumers’ behaviors.)
2. Understanding the existing ML/AI systems @ tiket.com
The tiket.com Data Science team has numerous ML/AI engines with functions ranging from recommendation to segmentation, detection, natural language processing, pricing, and prediction.
Because a majority of our earlier research pointed towards the direction of user-centered recommendations, we wanted to pick an ML/AI system that could help us better provide recommendations.
Simultaneously, we wanted to pick a system that hadn’t been translated in a design perspective for users to visually see and appreciate.
⚠️ Problem
1. Understanding current problems @ tiket.com
Hotel Funnel Analysis:
An analysis on User Retention and Drop-off Rates from the Search Results Page (SRP) to Purchase Form for Hotels
(Due to a Non-Discoluser Agreement, I’m unable to specify the exact numbers and percentage of users from this data.)
The biggest drop-off rate of users occured in the Room Listing Page.
Our team consulted the lead UX Researcher from this project to ask about any secondary conclusions or findings from the data and key points that we had originally overlooked were heavily correlated with what users did AFTER they had dropped off from that point:
Relevance Issue
Some users input the words “child” or “family” on the search form before they dropped-off.
Uncertainty Issue
Some users are uncertain about what they’re looking for and leave the Room Listing Page to change destinations on the Search Results Page.
Benefit Issue
Some users second-guess the benefits they’re receiving from the hotels they think about choosing and have the mindset that they might possibly find a better deal.
2. Empathetic Data Analysis: Breaking Down Information into User Insights
3. The ML/AI system we chose: Cygnus
Cygnus - Hotel User Segmentation
Categorizes users from multiple factors such as (1) click behavior, (2) transactions, (3) geolocation, and (4) their hotel booking process
To ensure accuracy and relevancy, the user segmentation models are run monthly, updating user clusters accordingly.
Although not directly related to user-centered recommendations, we decided that this aspect of understanding our users’ behavior and personality was an area of untapped potential for developing an impressive recommendation system.
🔍 A Look into Cygnus
Cygnus’ User Segments
Limited transaction frequency
Budget-conscious
Indifferent to Promotions
Indifferent to Credit Cards
Preference for Standard Hotels
Use of Budget Hotel Filter
Frequent transactions
Budget-conscious
Promotion-Aware
Indifferent to Credit Cards
Preference for Standard Hotels
Use of Luxury Hotel Filter
High-Spending
Promo Hunter
Moderate Credit Card usage
Preference for Luxury Hotels
💭 Our Proposal
Each user segment will have a different Search Results Page UI, with different recommendations specifically catered towards their interests and concerns.
Tailoring